Details
As businesses adopt generative AI, safety and responsibility need to be top priorities. This webinar brings together experts to discuss how AI is evolving, the biggest risks enterprises face, and what it takes to deploy AI securely. Panelists will share real-world examples, insights on stress-testing AI systems, and strategies for keeping AI aligned with business goals and risk policies. From adversarial testing to red-teaming foundational models, attendees will walk away with a clearer understanding of how to build AI systems that are both effective and trustworthy.
Speakers
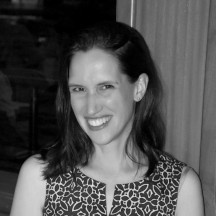
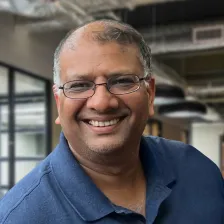
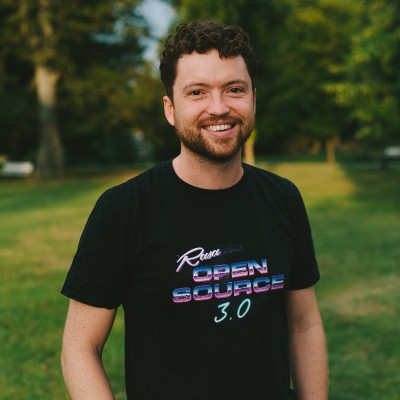
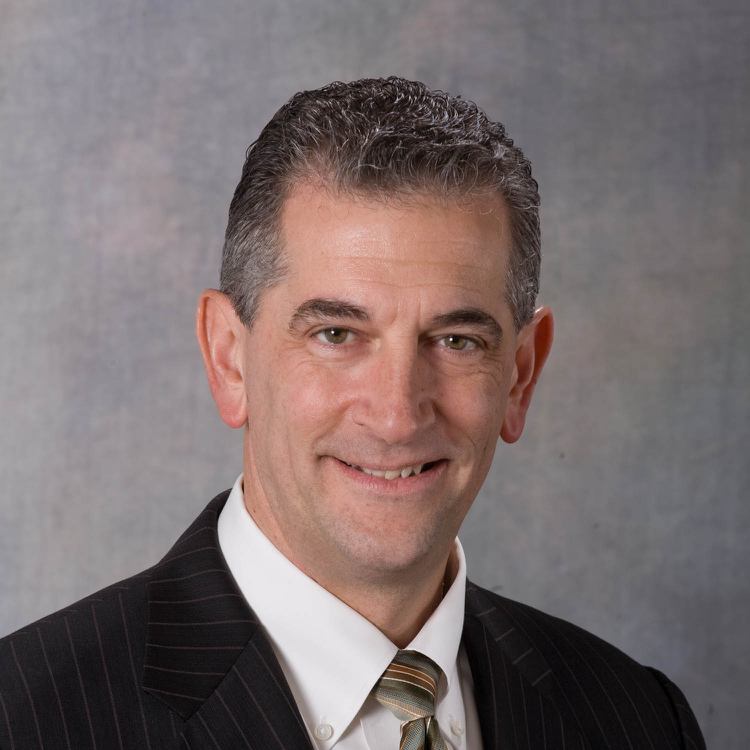
Post-event summary
The webinar titled “Deploying GenAI Applications Safely and Responsibly,” was hosted by EDM Council and Innodata and emphasized the importance of combining innovation with a strong framework for responsible AI development, particularly in customer-facing applications where risk, brand reputation, and compliance are top concerns. The webinar featured key speakers:
- Karen McNeil, LLM Red Teaming Practice Director, Innodata
- Rahul Singhal, Chief Product & Revenue Officer, Innodata
- Alan Nichol, Co-founder & CTO, Rasa
- Moderator: John Bottega, President, EDM Council
Alan provided a historical perspective on the evolution of conversational AI, from intent-based classification systems to today’s dynamic, LLM-driven interfaces. He explained the limitations of older systems that relied heavily on rigid business rules and stressed the value of combining traditional software architecture with the fluency of LLMs. Rather than allowing models to “run rampant,” Alan advocates for defining business processes clearly and using LLMs to handle nuance and variability in user interaction. “While the old style approach had too much structure, an LLM on its own has too little,” he said, pointing to the need for balance between structured and generative responses.
Karen defined the role of red teaming, a critical practice for identifying model vulnerabilities through adversarial and unintentional testing. She described how red teaming works in practice—simulating user prompts to expose model weaknesses—and explained that risks vary widely depending on the organization’s size, audience, and tolerance. Red teaming helps companies understand how their AI might react in edge cases, revealing gray areas that standard testing often misses. She also highlighted that models may behave unpredictably, especially when fine-tuned or integrated with additional data sources like RAG (retrieval-augmented generation), increasing the importance of continuous safety checks.
Both experts agreed that continuous monitoring and evaluation are essential, particularly as generative AI systems evolve in unpredictable ways post-deployment. Rahul outlined Innodata’s approach to building a continuous monitoring platform that auto-generates prompts and evaluates system robustness over time, akin to a “McAfee for Gen AI.” Alan emphasized that while fully automating safety evaluation is not yet feasible, organizations must maintain human oversight and build trust through structured processes and close data review.
The webinar concluded with calls for responsible innovation. Karen stressed that even models built on already-safe foundation systems must be retested if modified, saying that “fine-tuning can just destroy a model’s safety alignment.” John echoed the need for ongoing governance and human-in-the-loop strategies, reminding participants that effective AI oversight must extend beyond deployment.